Getting Philosophical with the Internet of Things (IoT)
IoT with Robert Welborn: Aristotle
Add bookmark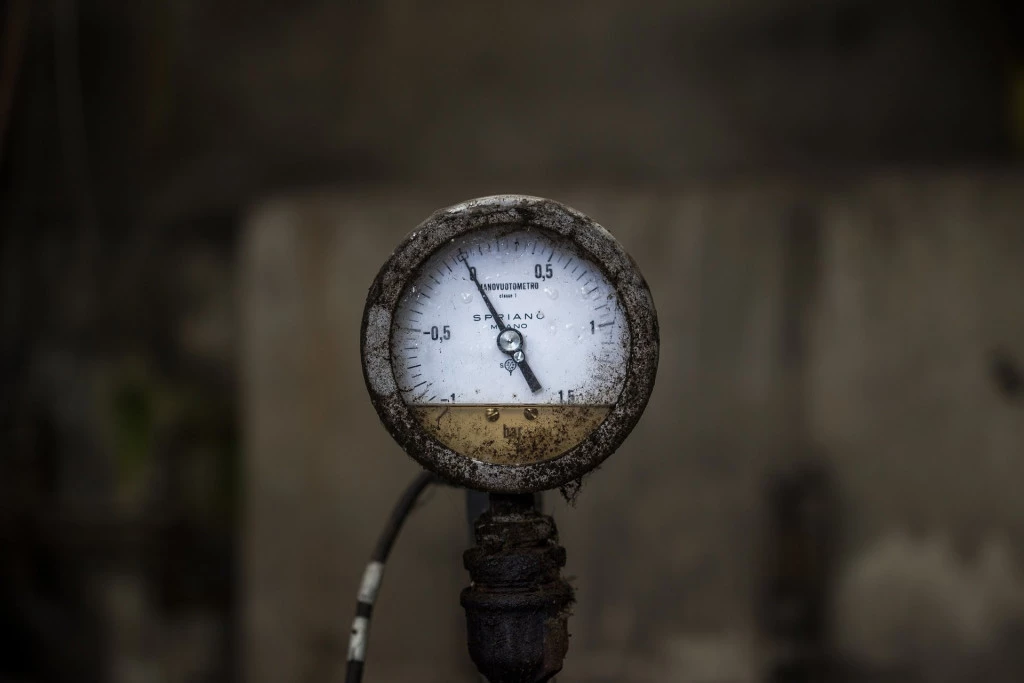
The Internet of Things (IoT) was formed as a way to answer questions. When an engineer needs to know how something works—or why it doesn’t—installing sensors as rudimentary as the thermometer helps answer these questions. Sensor technology has evolved with technology at large. First it became decipherable, translating into dashboard lights and readouts. Eventually it became wireless, showing up through virtual dashboards via the internet.
Sensors are all around us, and they’re designed to transmit a particular piece of information. The Internet of Things enables those sensors to feed into a system that looks at data holistically, creating a user experience that goes beyond singular datasets. For example, IoT technology not only reads the temperature of your car; it knows when you turn your heat up and down, and it eventually learns your temperature preferences and adjusts accordingly. If your smart thermostat at home records these same preferences, your entire connected environment is able to adapt to your preferences. This is the power of the Internet of Things.
The Law of Identity
Aristotle tells us that A is equal to A. That is, a tree is equal to a tree. He further explains that once you’ve cut down that tree, it is now a log. Carve up that log and you have a board. By shaping that board with intention, you've created an arrow.
Energy is exerted with each transformation. In the case of the arrow, both mental and physical energy went into the transformation. What we have left is something new. An arrow is not a tree. They have something in common, but energy has transformed that thing. As Aristotle put it, that tree’s potential has been manifested into a new existence. After all, an arrow makes a lousy shady spot to contemplate the universe, just as a tree doesn’t make a very good hunting apparatus.
The Law of Identity: IoT Edition
As the tree is raw material transformed into an arrow through energy, so is sensor data. It isn’t until energy is added to it that it becomes something new. The power of IoT doesn’t lay in the data alone. That data has already been used.
We already know how hot the thermostat reads. In order to derive new insights from that data, we must add energy back into it. In the case of Internet of Things, we’re adding math and computing power.
For example, if we pose the question of whether or not SUV drivers are consuming or burning more carbon than battery electric vehicle drivers, temperature sensor data alone doesn’t help us out much. That data already told us how warm parts of the car are. In other words, it’s used data.
However, if I take those temperature readings and turn them into a temperature model, then I use that temperature model and cross verify it with experiments with other temperature models, I now have an idea of which temperatures are predictive of energy use. I’ve answered the question of which temperatures are particular to SUVs versus electric vehicles. Old data and energy in: new data out.
It’s a fallacy to believe that you can take legacy systems, legacy sensors, and harvest usable and/or sellable IoT data as-is. As Aristotle taught, we need to add energy to transform it into something new.
Models and Model Scores
The input of the math energy involved in creating something new results in a model output. For the sake of comparison and usability, each model has a score. A credit score is a perfect example of a model score. We know—and creditors know—we’re sitting pretty if our score is hovering around 800. If our score is around 340, that paints another picture entirely. Although the math behind that score remains a layperson’s mystery, we understand what the model represents.
The stock market works the same way. If the S&P is up 100 points one day, we know Wall Street is popping the cork.
Building and working with models gives us a quick, self-referential means of understanding. We call a tree a tree because we’ve defined the model of what a tree is. An effective model does the work of uncovering and conveying insights for us. As we share and spread these insights, voilà. With a little bit—or a lot—of energy, the old data gets recycled into something new.
In terms of the Internet of Things, we can think of that old data as digital exhaust. It’s been used. It is only through the recycling and reshaping of that data have we created something innovative and functional.
The Problem With Big Data
What some fail to understand is that the vast amounts of data being collected has already been used or is in its raw, unused form. Raw data goes unused because installing the sensor technology comes first. Models can’t be created without data, so for the sake of potential future use cases, sensors are programmed to collect data of all kinds. Whether or not that data gets used is dependent on the insights we wish to garner and the models we create to achieve that.
Data alone doesn’t solve any new problems. The data collected is the output, or exhaust. New insights get created from that exhaust via models and experiments. With IoT, as with many other models today, these models are artificial intelligence (AI) models.
Data Collection in Action
There are 350 million of us, and while we are all unique, our data is not. This is good news. When our personal data is collected legally and ethically, it simply goes into a pool of others’ personal data. The goal here is to get a big-picture view of the way humanity—in its most general definition—thinks. This data goes into models that look at us as a collective, not individuals, for the sake of telling an actionable story. Data collection done right isn’t a breach of privacy, but a practice that has withstood the test of time—just supersized.
Technology has allowed researchers to do what they’ve always done, albeit on a much larger scale. They’re still taking a sample of a population, comparing it to another sample, and proving that sample A is different from sample B.
Telling a Story With Data
Now we have data, a model for that data, and insights from that model. So what? The value that energy only comes when we decide to do something with it. Do we use it to build new parts, build better cars, build better garbage disposals, build better thermostats? Or do we simply package it up and sell it?
I like to use the pothole example, which goes like this: A car drives over a pothole, and the sensors in that car deliver data to the models we’ve created, which deliver a latitude and a longitude and the probability that there's a pothole there.
The raw data is going to be the latitude and the longitude, and then a whole bunch of telemetry settings that tell me how much the wheel slipped, how far the wheel compressed, how far the spring compressed, how rough the engine felt when it went over it. It's a whole series of these things. None of these data points tell me the probability that there's a pothole there. The model takes all of those things together and gives me a model score. In this case, the probability of a pothole.
Finally, a pothole filler is going to look at those insights and decide whether or not to take action. Model scores are only as useful as the actions taken on the story they tell.
Looking into the Future
Forward progress is the natural plight of humankind. As Aristotle said, “The energy of the mind is the essence of life.” IoT is harnessing this energy into the next industrial revolution limited only by our imagination.