A Quick Guide to AI in Life Sciences
Influencing consumers of the healthcare and pharmaceuticals industry products and services
Add bookmark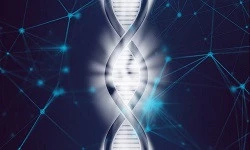
A Quick Guide to AI Life Sciences
From sea to shining sea—and above and below—nature created organisms so vast and varied humans are still discovering them. Even within our own bodies and brains, mysteries abound. While medical advancements have improved our quality of life and saved us from some of the awful fates of our predecessors, we still have a long way to go.
With the advent of cloud computing and big data, artificial intelligence is beginning its foray into life sciences. It is advancing the way we diagnose and treat disease. Roadblocks that plague medical research are being cleared by AI. By combining the greatest minds science has to offer with the computing power of AI, our understanding of the complex systems that are the life and death of us grows.
Although the adaptation of AI in life sciences is slower than in other industries due to the regulatory landscape and mystery behind AI’s “thought process,” the general consensus across the industry is that AI is the way forward in life sciences.
Diagnostics
Deep learning is the subcategory of AI that processes hundreds of thousands of labeled data points, recognizes their patterns, and can then draw accurate conclusions on unlabeled data. In diagnostics, AI is able to screen for cancer, eye disease, and childhood illnesses with the same accuracy as trained professionals. Diagnosing backlogged medical images and providing expert screening services in medical deserts means diseases are identified and treated sooner, leading to an increase in positive patient outcomes.
Cancer – AI enables improvements in cancer diagnostics. The application of AI in cancer detection uses computer vision to spot and differentiate between malignant and benign masses. Tumors change the behavior of the affected tissue. By feeding an algorithm labeled images of these tissues, it is possible to train the algorithm to correctly identify masses in unlabeled images. Access to electronic health record (EHR) data moves the needle forward in accurately training these algorithms.
An early diagnosis is imperative for the successful treatment of cancer, and the power of AI can detect subtle abnormalities that may be missed by routine scans. However, research into this realm is still in the early stages and presents issues to overcome. The way AI learns exists in layers. The middle layer, known as the hidden layer, is still a data science mystery. Some believe that doctors can trust the results of AI algorithms only after the black box of its decision making is unlocked.
Cardiology – Heart disease is the leading cause of death worldwide. Echocardiography takes images of the heart that are interpreted by doctors to diagnose heart failure and other circulatory issues. However, disease identification in cardiology takes a trained expert with many years of experience and is subject to several variables.
Recently, the FDA took a big step forward in the detection of these issues by approving EchoGo Core, an AI powered echocardiography analysis program. This exciting advancement means early detection of heart disease is more attainable than ever.
Genomes – The human genome consists of over 3 billion genetic letters. While we’ve come a long way in the sequencing of genes that create a roadmap of our DNA, we haven’t made a lot of inroads regarding where that information takes us. Genomic sequencing has suffered from data overload. Sequencing alone isn’t enough to improve human health.
AI systems are being trained to process this massive amount of data to spot correlations in genomes and disease—a task that could take several lifetimes for humans to sort out. That means genetic screening of expectant parents can predict genetic abnormalities. It also means the exact variant of a genetic abnormality in DNA can be pinpointed, which aids in gene therapy or even the application of the revolutionary—albeit controversial—gene-editing CRISPR technology. But that’s a story for another day.
Preventative disease management
Chronic disease affects about 133 million Americans. While these ailments have no cure, managing pain and making lifestyle changes drastically improves the quality of life for this 40% of the population.
AI is changing the way diseases are managed. Patient data is vast and ever-changing. Every time a person sees a doctor, gets a vaccination, or adds a supplement to their health regimen, their patient profile changes. Thus, it is easy for patients to fall through the cracks and miss out on critical personal preventative and maintenance care. The computing power of AI is able to pore through patient medical records in a fast, reliable way, flagging potential treatment therapies and missed opportunities for chronic disease patients. Additionally, 80% of chronic care patients drop out of their therapeutic programs for a variety of reasons. AI looks to change that.
Wearables
Promising AI pilot programs are rolling out across the globe. They involve providing wearables such as armbands that take vitals remotely, including pulse, blood pressure, heartrate, and oxygen levels. This data gets processed by AI in real-time. It is then crosschecked with global patient data, which leads to early detection of disease complications and a decrease in hospital readmissions.
For example, patients who suffer from stroke and seizure disorders stand to benefit from AI wearable technology. AI, and more specifically, ambient intelligence, (AmI) help monitor and predict complications in neurology noninvasively and inexpensively. Stroke and seizure treatments are best effective when administered immediately. However, the early warning signs of an attack are easily overlooked, and by the time a patient recognizes the symptoms, they may be too incapacitated to seek treatment. AmI wearables monitor neurological functions around the clock, spot discrepancies and abnormalities, and can even alert emergency medical services.
Clinical Trials & Medical Research
Cancer death rates have dropped by 27% in the last 25 years, in part due to early detection and more advanced treatment options. However, the road to new clinical therapies across the spectrum of disease is arduous. Trials suffer from a number of bottlenecks. Rare diseases don’t receive the funding necessary to search for cures. Patient populations aren’t always large enough to fill a trial. The potential for AI to solve these problems means life-saving therapies can be quickly and affordably researched, approved, and prescribed.
Recruitment
One of the biggest barriers to success in clinical trials is recruitment. The branch of AI called natural language processing (NLP) works to solve this. NLP understands both oral and written words. That means NLP algorithms can process patient databases that include pathology reports and doctor’s notes to seek out eligible trial participants. Finding eligible clinical trial patients takes minutes, not months, with AI.
Patient Monitoring
While AI technologies have only assisted in clinical trials in the past five to eight years, these trials are adapting to the power of AI in several ways that benefit the study. Clinical trials often fail because the burden of labor lands on the patient. They are responsible for manually tracking and recording vitals, medicines, and activities. Because of this, 40% of patients don’t stick to the trial protocols within the first 150 days. Even worse, many drop out. Patient adherence is easily trackable in real time with AI wearables and offer built-in reminders.
Additionally, trials are complex and involve a lot of data. With AI, and in particular, machine learning, natural language processing, and deep learning, trials benefit from the processing of data in a fast and reliable way. Through automatic patient monitoring, adherence improves which improves the accuracy of end data points.
Regulatory landscape in AI life sciences
AI is advancing quicker than regulations can keep up. It will be some time before the full benefits of AI in life sciences are realized. For example, a treatment that utilizes an AI algorithm must first be approved by the FDA. However, the algorithm is required to remain stagnant after approval. This means it can’t benefit from the adaptable power of machine learning without going through an entirely new approval process.
Further, regulatory transparency is mission critical in drug development. If AI makes conclusions that reside within a black box, no matter how accurate or life-saving they may be, they aren’t capable of being peer reviewed or properly retested. Some scientists believe this black box bias is outdated and irrelevant—that if a therapy works, it works. Others worry that the black box will leave therapies prone to bias and discrimination. Regardless, FDA recognizes that their current model is unsustainable for the future of medicine. However, historically, the FDA is a slow-moving ship, and changing course will take time.
Conclusion
AI in life sciences offers exciting potential for the prevention, diagnosis, treatment, and perhaps even cure for disease. The layperson will benefit from AI in the form of wearables and increased control over their personalized health. Custom, targeted treatment plans are easier to draw up with AI, as no patient data falls through the cracks. Lifestyle changes enabled by AI wearables increases the prevention of disease and improves overall health in a sustainable way.
AI is also the future of discovery regarding the intricacies of the human body and the diseases it’s prone to. The ability for AI to process vast amounts of data faster than a team of data scientists and doctors means that human talent can be funneled into the invention of new pharmaceuticals and therapies at a lower cost and shorter time-to-market than ever before.
References
Davis, N. (2019, September 24). AI equal with human experts in medical diagnosis, study finds. The Guardian.
FDA Clears AI-powered Cardiac Echo Analysis and Quantification by Ultromics. (2019, November 14). Diagnostics and Interventional Cardiology.
FDA's Evolving Regulation of Artificial Intelligence in Digital Health Products. (January 2018). JonesDay.com.
Guest, A. (2019, March). Current remote patient monitoring reduces readmissions at Dartford and Gravesham NHS Trust. Health Tech Pulse.
Heath, S. (2017, June 9). Overcoming Patient Barriers to Chronic Disease Management. PatientEngagementHIT.com.
Kent, J. (2019, July 18). Artificial Intelligence Could Increase Clinical Trial Success Rates. HealthITAnalytics.com.
Kuan, R. (2019, October 18). Adopting AI in Health Care Will Be Slow and Difficult. Harvard Business Review.
Schneider, N. (2019, September 19). Artificial Intelligence – Improving How We Diagnose Cancer. TechnologyNetworks.com.
Simon, S. (2019, January 8). Facts & Figures 2019: US Cancer Death Rate has Dropped 27% in 25 Years. Cancer.org.
Weintraub, K. (2019, August 13). Despite Controversy, Human Studies of CRISPR Move Forward in the U.S. Scientific American.