The boring data conversation
The Ether w/Casey Simple: On Data
Add bookmark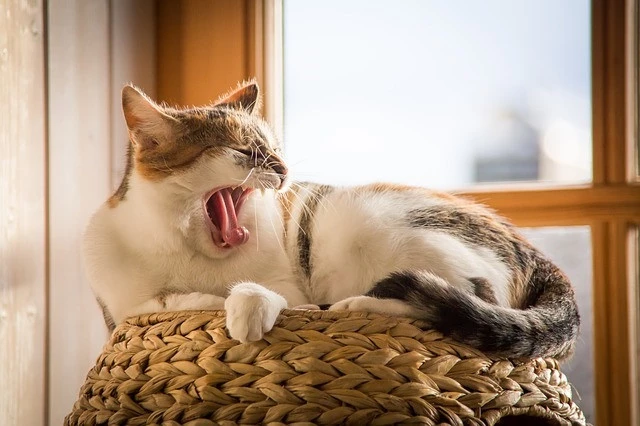
In this installment of The Ether, Casey Simple explores data:
The world of AI exciting. The data maintenance behind that world is, quite frankly, boring. We’ve been dealing with data for decades. We’ve maintained it, cleansed it, and analyzed it. Following AI’s long winter(s), enterprises are begining to truly transform.
Now, we’re using applications to systematically examine data across multiple departments and multiple systems. We can now analyze data at incredible speeds, which has further pushed the data topic forward. We’re taking our data systems of the past and reinventing, recycling, and revamping them to be applicable in today’s AI environment.
We must be careful about how we combine this old world of data with the new. There are three key essentials to setting up a solid, systemic focus on data maintenance.
Assign Data Owners
A data owner is responsible for understanding specific data elements within a well-defined category of data. They know the granular details of access policies, issues with source data, and processes for using data. A data owner is separate from a process owner or an analytics owner. They are focused solely on specific data ownership and can answer questions like: How do you maintain data? Why is data maintenance important? Why and how do you disseminate data elements?
For example, a revenue data owner oversees all the data elements that feed a revenue algorithm. Similar data shows up on reports as well, so we may already have some experience with it from an analytics perspective. However, a data owner examines this data from an AI lens and focuses specifically on the algorithm at play. This might include data from ordering systems and accounting systems.
The owner identifies who can see this data and at what times during the accounting cycle, which is especially critical in terms of revenue data in a public company. They look at any use of the data elements or algorithms that are calculating revenue, and they are responsible for person who reviewing and approving this particular use of it. They are also tasked with maintaining consistency companywide so that any time revenue data is used, an algorithm is always defined the exact same way. Ensuring the algorithm’s consistency means it isn’t being defined as something different in different places at different times.
Additionally, it is possible the owner is working with the accounting team to look at practices and policies such as revenue deferrals to access any changes in that policy that might affect the algorithm. They can also suggest processes for routine maintenance and cleanup of deferrals on a predictable schedule to maintain consistency in how revenue is being used in an algorithm.
Create a Data Maintenance Team
A data maintenance team is in charge of complex and multisource data. Customer data maintenance teams are a common use case, as customer data is vast and comes from every direction. If an organization assigned a data maintenance team during a previous transformation in shared services, it makes sense to leverage the same team. However, it is necessary to redefine the scope of the data maintenance team to fit with the AI environment to ensures long-term success and adaptation.
A data maintenance team is looking at source data and determining where it comes from, how it compares with existing data that’s been cleansed and maintained, and determining whether or not it should be added automatically. Adding new data without scrutiny creates a host of problems. While real-time data is desirable, clean data is imperative.
It’s a balancing act for a data maintenance team to decide what new data needs to be cleansed before it is added—if at all—how much it needs to be cleansed, and if it should be merged with existing data. There is also the question of what data is better, and how to avoid duplicate data.
A data maintenance team also works as a companywide data desk. A data desk becomes a destination for any other internal entity who questions some of the data they’re using in an algorithm and wants it changed, validated, or cleansed. Using customer data as an example, legal entity names or tax information changes all the time. This type of data shouldn’t be changed within a system in silos or on the fly. A data maintenance team ensures the integrity of the underlying data.
Prioritize Data
Data owners and data maintenance teams aren’t going to be productive if they’re mired in lower-level, less impactful data. To figure out what data deserves precedence, look at the top five reports your c-suite receives and uses regularly. Generally speaking, the data on those reports is similar to the data utilized in the most frequently used algorithms. The data elements in those algorithms are also worth a look.
If you assign ownership and maintenance to those two things, you know you’re working on the most critical data for the company. Begin with that. Its impact on setting up a nice, boring routine will lead you to long-term success around your AI environments.
Conclusion
In the case of data, the opposite of boring is chaos. By setting up the right framework from the get-go, you’re saving time, money, and an endless amount of stress and frustration.