State of AI & Intelligent automation network report
Report of AI & IAN state in the market
Add bookmark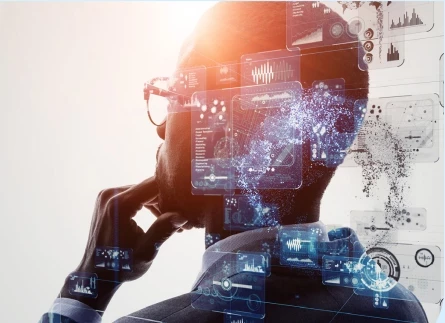
AI & Intelligent automation network
It has become ever clearer that corporate enterprise practitioners must scale intelligent automation. You must also re-establish whether or not you’re on the right path as you plot your course on your intelligent automation journey. But you can’t fall behind, so you’ve got to start working with cognitive solutions now. Next generation solutions thirst for your unstructured data — but, by definition — you’re having a tough time identifying that data. And even if you could — those cognitive solutions must learn from your current infrastructure, which needs systems integration. And wasn’t this supposed to be something you could do without IT?!
The AI & Intelligent Automation Network feels your pain. And so, we’ve asked friends and community members to not only lay out what they’re doing but to provide some feedback on what you can do right now to transform your enterprise before it’s too late.
The key for global corporate enterprise is to benefit from the collective intelligence presented by RPA and cognitive technologies along with human workers. Only by having technology combine with human talent can global corporate enterprise achieve scalable intelligent automation. And only with scalable intelligent automation enterprise resiliency be realized. Join that community for lessons learned at SRIA Live.Scalable RPA & Intelligent Automation Live
• Nadia deVilla shares thoughts on re-engineering the enterprise through metrics
• Tony Saldanha discusses realizing 5 step-changes to digital transformation
• Cindy Gallagher urges you to really deal with your carbon-based workforce
• Rohit Amberker advises that exponential impact has arrived, so we must now act accordingly
• Marcin Nowakowski believes that automation scaling up will come through agile transformation
• Manish Rai unpacks your dark data to democratize your digital transformation
• Lee Coulter wonders if we’ve got the talent to do this while laying out how we can do this
• Jerry Wagner has discovered a metric methodology, which informs the enterprise
• Bob Kurpershoek shares ideas on building your team to tackle dark data now
• Rajeshwari Ganesan provides insight into the design of the human brain
• And Aric Dromi does his best to think differently about the larger issues at hand
You’ll notice that we don’t have a ton of statistics in this report. That’s by design. These pages feature your colleagues speaking your language and providing actionable takeaways. Besides, we’ll be providing new market statistics in our next report: AI 2020 — our annual update out in January 2019.
They say the Future is Now — it’s a big concept — it may mean carpe diem. For corporate enterprise practitioners it just means very hard work that needs to be done in a new and different way. Sure, you can learn from the past — but one of the keys in these pages is to unlearn from the past as well. Here’s to your mind.
One small transformation for me, one giant transformation for the enterprise
A review of the landscape when I arrived
Two and a half years ago, Manulife was in the midst of a strategic crossroad. The organization knew it was ripe for change and the lack of any bold action would trigger their decline.
Also read: What is Process Mining?
“Transformation” was more than a buzzword for us, and investments were poured into a much needed, large-scale, multi-year technology overhaul. However, this consumed business owners who in-turn were mostly overwhelmed as they attempted to balance business-as-usual with transformation.
I had the good fortune of joining the team whose mandate was and is “to drive Business Optimization within the Investments Division of the organization.” But with a mandate like that, where does one begin?! How does one optimize when the business is being turned on its heels?!
We initially started by taking on a logical yet huge task – help businesses redesign their target operating model.
We had some successes; however, businesses could not think beyond their current challenges. We pivoted and took on tactical work (i.e., writing procedures). We then extended our efforts by taking on the responsibilities of preparing businesses for the change (i.e., organizational readiness). This allowed us to get entrenched within the businesses while allowing us to become more relevant to the business, albeit tactically.
Leap forward a few months, with a couple of conversion phases under our belt — our team was able to highlight and identify gaps within the transformation (i.e., proliferation of manual procedures despite introduction of technological changes). Because of this, we were able to get seed funding to conduct an RPA pilot to determine how we could accelerate or augment transformation and eventual benefits realization.
What we started and how we measured it
We had a successful pilot where we were able to demonstrate and measure the capacity lift created by robotics. The ability to measure this earned us the opportunity to extend our efforts. A key learning from the pilot as well is the importance of having a tool to measure (even at a high level) the effort and benefits of applying robotics onto a process. With a simple excel tool and straightforward criteria (such as time spent, level of resource, structured/unstructured data, number of systems in use), we were able to measure complexity and feasibility of robotics.
This allowed us to have objective conversations with the business owners and allowed us to demonstrate how we can augment existing transformational efforts – that is, we were not bastardizing the benefits from the large-scale program, rather accelerate if not add to it.
The digitaliztion & automation item approach and resource capabilities can be expanded to other innovation technologies.
What we changed and the benefts and value received
Key ares we affected were:
1. How we viewed manual procedures – i.e., these are gold and a feeder to our robotics efforts
2. How we viewed our captive outsource in Manila
Benefits and values received:
1. Standardized processes – enabled us to consolidate work within teams and drove to more standardized processes and limited exceptions
2. Low-cost technology – allowed us to introduce automation changes much faster 3. New capability / skill for the team
How we're now adding value to the enterprise
In addition to the above, we were able to reposition and even rebrand the team to the Digitization and Automation team. In addition to Robotics, the approach and resource capabilities can be expanded to other innovation technologies. The team is now positioned to continue to add value by not only enabling the organization to meet its financial targets, but we are now our enabling the organization to think innovatively and enable them to fail quickly, yet safely in the quest of doing things better.
And that one step for me:
I personally have now moved on to head our digital transformation efforts within the insurance division.
Transformation with tony
Why most digital transformation goals wrong
In his second column for the AI & Intelligent Automation Network Tony Saldanha explains that we need a common definition of true digital transformation; something that distinguishes the rebranding of old technology and process improvement efforts from a failsafe pathway to a new enterprise reality.
OK, so I realize that the headline is provocative. I seem to be condemning a lot of smart leaders who have been courageous enough to set up transformational goals in the first place. My apologies to them; I don’t mean to be an arm-chair critic. My intent is to take aim at the tendency to recast traditional automation and process-change work as digital transformation. That’s just a destructive tendency that causes a lot of damage all around. Let me explain.
If you cannot lock in on the true definition of transformation, how do you expect to get there?
Here’s the dirty little secret of digital transformation. It’s a term that has been hijacked by every technology and consulting provider and IT professional with an agenda. If you cannot lock in on the true definition of transformation, how do you expect to get there? There are pitfalls everywhere, starting with the confusion between digitalization (which is the use of technology to automate work processes, using say, SAP), and transformation (which is creating dramatically new value for customers via say, entirely new business models). Then there’s the issue of anecdotal successes at the fringes of the organization vs. systemic cultural change using digital technology.
As a result of this issue of defining true “digital transformation”, most business leaders become frustrated with the whole topic of digital disruption. It seems to be a moving target. They have invested serious effort and money because they know it’s an important topic. Technology -savvy competitors are already de-stabilizing their existing business models. But, despite the best efforts of their organization - and several anecdotal successes, they’re clearly not at the end of the journey. In fact, they’re not even sure they know where they are on their journey, or how long that journey will take.
The clock of digital disruption keeps ticking inexorably towards business model obsolescence
Additionally, this can be a corrosive issue for business survival in the digitally disruptive era. Rebranded digital activities can generate a false sense of confidence among business leaders - that what their organizations are executing is digital transformation. Meanwhile, the clock of digital disruption keeps ticking inexorably towards business model obsolescence.
We need a common definition of true digital transformation; something that separates true digital transformation from the rebranding of old technology and process improvement efforts into the latest buzz words. We need a failsafe pathway to the ultimate stage of digital transformation. I call that Digital Transformation 5.0.
The Digital Transformation 5.0 model puts the journey into context and provides a deliberate roadmap to recognize and move up the stages of transformation including:
· Digitalization: Getting the scaled benefits of automation
· Opportunistic: Departmental benefits of disruptive models
· Synchronized: Critical mass of corporate disruptive wins for customers
· Migrated: Big competitive win for the moment
· Digitally Native: Ongoing, sustainable win versus your industry
True Digital Transformation locks its sights on Stage 5 i.e., getting to be a “Digital Native.” It is truly transformational; in line with the fact that the current Fourth Industrial Revolution demands entirely new digitally based business models. It’s sustainable in terms of changing the culture of the entire enterprise, so that operating as a digitally native enterprise becomes second nature on a going basis.
So, what are the “right” goals for business transformation? Firstly, they are not just about one-time project success, but conceive of sustainable change.
Secondly, they need to address the following questions.
• Has digital operation become your DNA?
• Are you able to perform as an ever-evolving market leader?
• Are you able to provide hugely personalized, creative value to customers?
• Do you have among the most innovative business models in your industry?
• Will you be able to sustainably stay on top because you’re continually innovating in a disciplined way?
Is that a high bar? Absolutely. But this is what it takes to survive an industrial revolution!
Transforming a digital workforce as a practitioner CEO
In this headlong rush to intelligent automation, there is more opportunity than ever to layer judgment-based, culturally empathetic work on top of process automation. Short-term wins will be achieved in automating those process steps, which are cumbersome, transactional, and error prone. However, the greatest long-term return for any automation endeavour will come from analysing a process end-to-end and repositioning the human workforce at points where judgment and empathy are required.
Corporate executives are engaging with automation opportunities as a method of controlling cost, improving control and enhancing customer service. The most innovative of these executives are able to harmonize their talent development initiatives with their automation initiatives. They understand the need for a dual transformation strategy; one, which focuses on the digital workforce, and one which cultivates the power of the human mind.
The reality of the digital industrial revolution we’re in right now, isn’t that jobs will be totally lost — it’s that jobs will change. Jobs will change to such a degree that not every individual will be able to adjust on their own. Leaders must take as much care of planning for the future of their human workforce as they are of their digital workforce.
At the most basic level, someone must build and care for the digital workforce. Leveraging automated intelligence, the digital workforce will be able to teach itself to some degree - but only a human can get it to that point. As much as we’d like to believe that the digital workforce never gets sick, it does. When it does get sick, through a virus, a hack, or any other means, the best solution today is for a human to care for it. In a way, the digital workforce needs a healthcare and leadership structure of its own.
Secondarily, there will always be the need for human-to-human interaction. Cultural, empathetic and judgment-based work still requires human interaction. The digital workforce can’t yet understand empathy. Bots can’t sit across the table from a customer, look in their eyes, understand the customer’s feelings or take note when body language gives clues of something unsaid. And intelligent automation of any kind simply cannot express the level of trust stakeholders demand and deserve. Leaders must help our human workforce understand how to communicate with stakeholders on a new, deeper level.
We have no choice but to embrace the digital transformation happening around us. The changes in our industry are exciting and happening at an exhilarating pace. If leaders slow down, or try to deny it’s happening, they will be left behind. If they try to embrace only the digital transformation and forget about the human transformation that is happening at the same time, they will be left behind.
To truly transform operations, we are required to have both a digital workforce strategy and a human capital strategy. The two must be harmonized with aligned milestones and clear expectations. If done correctly, corporate executives will achieve their goals of cost reduction, control and customer satisfaction, all while gaining the trust of their team members and their clients.
Exponential impact has arrived, act accordingly
All research and market studies more or less conclude
1. Digital transformation is occurring at a pace never seen before. Technology and digitization is impacting every business from restaurants to grocery stores to cars.
2. Jobs are getting disrupted and new job categories are evolving in everything from manufacturing to autonomous cars and trucks to accounting
3. Very powerful technologies are emerging such as AI / ML, Robotics, Blockchain and they are already proving to be disruptive
4. The impact of all these technologies is exponential. A whole industry can be created or destroyed. Uber created a completely new industry; warehouse robots are completely replacing warehouse jobs.
Also read: Workload Automation
Realities of today
Digital Transformation:
No matter how you define it, enterprise, business, function, leader and worker all must aggressively transform to be in a position to benefit from artificial intelligence
Cognitive and process jobs disruption:
Enterprise, business, function, leader and worker must work towards that new paradigm today
Emergence of powerful technologies:
AI/ML, Robotics & Blockchain have already disrupted the enterprise.
Exponential Impact: Unlike ERP, Cloud & Mobile, AI does not offer iterative change
Studies indicate accountants and auditors have a 93.5% chance of being automated and hype-cycle news remains in full effect:
Digital business create more value and market rewards digital businesses. Unless traditional businesses digitize their operations, they will soon become irrelevant.
Digital businesses create more value
Bots are clearly taking over manufacturing jobs and even with reduction in jobs, the manufacturing output continues to grow significantly. This is due to introduction of bots in the manufacturing industry.
The direction of economic growth
We are focused on process automation, anomaly detection, customer service and leveraging our known and dark data.
Applying Technology to Finance Operations Automation
“The first rule of any technology used in a business is that automation, applied to an efficient operation, will magnify the efficiency. The second is that automation applied to an inefficient operation will magnify the inefficiency.”
~ Bill Gates
The numbers are in on where automation is having an empirical effect
Trends
1. 20 percent - 30 percent of Procure to Pay jobs are automated
- Invoice Processing
- T&E
- Payment Processing
2. 20 percent - 30 percent of Order to Cash jobs are automated
- Collections
- Billing
- Revenue Reporting
3. 15 percent - 25 percent of Record to Report jobs are automated
- Analytics Reports
- Fixed Assets
And most importantly, the numbers are in on where job growth will occur. The industry already has a shortage of talent in BI, ML, Logic Reengineering and good old fashioned customer service:
- New In- demand Skills For F & A Jobs
- Technology skills such as RPA, POwerBI, Machine Learning. R, etc.
- Customer Obsession and Customer Empathy Skills
- Problem solving skills for bot Process and IT
- Process + Logic Reengineering skills
Change in skills demand and composition
The tectonic plates are certainly already shifting. But the solutions have already made themselves apparent:
• The fact that digital businesses create more value can be proven to Senior Management
• Macroeconomic forces already showcase bot vs. carbon-based growth
• There are proven case studies of how and where to make automation work in global corporate enterprise
• The need for jobs of the future has already arrived
• Build your enterprise accordingly
Scaling automation through agile outsourcing
As you are reading this piece — every few minutes — a project is kicked off to automate processes in a particular outsourcing organization. Vendors are preparing sales pitches, operational teams are choosing processes to be automated, and the outsourcing world is evolving. What will be the result of this evolution?
I strongly believe that we are missing the honest, fact-based and open debate on the impact of automation on the outsourcing ecosystem. Yes, we’ve all seen headlines like, “Robots Will Cannibalize Your Jobs” and “99 percent of Accountants Will Be Jobless.” And we’ve seen provider sales pitches, “RPA Will Allow Humans To Do More Human-Related Jobs.” But each of those standpoints are biased, one-sided opinions - either too optimistic or too gloomy. Therefore, if debate is missing there is no better way to react than to start it.
Fact number one is that future of the industry is inevitably connected with digital revolution. The basic result being that organizations will need access to specific hardware and software but primarily need access to specific upskilled digital transformation talents. Those talents (executing professions - which might not even exist today) will evolve around digital tools and specific, automation-related change or transformation methodologies. Even today, at the early stage of automation, the available pool of those talents is very limited. Soon organizations will learn that the only way to get those skills is through “compact outsourcing” or “micro-outsourcing.”
Fact number two is that unprecedented velocity of change, which is happening in and around outsourcing industry, will force the ecosystem to search for solutions. The ecosystem must manage constant change understanding that there is a shortage of talent who can quickly develop the internal digital skills needed for true enterprise agility. The current approach of “develop internal skills” versus “find a big vendor” will not be enough to digest the amount of transformation required.
Fact number three is that while creating posts for digital and transformation talents, automation will gradually cut the jobs from the low end of the pyramid — manual, repetitive tasks. Although a lot is said about need of “up skilling” and preparing the workforce for the digital revolution, not much is visibly done on the actual ‘battlefield.’ Governments, outsourcing organizations, corporations nor employees are kick-starting the “digital upskilling storm.” And having in mind the size of the industry, “storm” is the most appropriate word that comes to mind.
Those three facts — difficulty to acquire people having relevant digital or transformation skills, unpreceded velocity of change in the industry, and decline of manual repetitive tasks will — as a mix with other factors of the digital revolution — bring different results for different geo–zones, industries and employee groups.
Service process outsourcing will be (is) highly prone to this “change cocktail” as it is built around repetitive tasks which are prone to be best delivered and organized in big groups of employees. Geo-zones housing most of outsourcing industry will see an impact of a different scope to the geo-zones housing digital revolution resources.
As you are reading this article, every few minutes, a project is kicked off to automate processes in an outsourcing organization. Vendors are preparing sales pitches, operational teams are choosing processes to be automated, and the outsourcing world is changing. What should be our answer to that?
Unpacking your dark data to democratize your digital transformation
The first wave of automation in enterprise has been focused on structured processes; repetitive tasks, they follow the same path, using structured data. That has brought tremendous value to enterprises. But only about 20 percent of the data in a given enterprise is structured. 80 percent of the data is unstructured. We’re talking over here documents, such as invoices, market applications, and explanation of benefits. Emails coming in stating, “What is the status of my invoice?” or “What is the status of my order?” That’s just the beginning of your unstructured notes and service tickets.
And there’s where the market is headed. The next level of value is being derived by attacking the semi-structured data of this tremendous opportunity around documents such as invoices and POs. Sometimes invoices and POs represent close to 50 percent of the volume of documents inside enterprises. So, we are seeing a tremendous amount of traction around structuring those documents.
The next thing I think we’ll see next year is not only in solving unstructured data but looking at the other dimension — making unstructured decisions. Unstructured decisions are predictions made after observing humans and the sequencing of tasks. Cognitive technology will be getting to a point where it’ll be able to predict the next best action and recommend the next best action. Cognitive technology will be able to take data from decisions people are making. Think about decisions people make around, approving market applications for example. Once the cognitive technology has observed hundreds of those decisions, it can build a model and start recommending whether the market application should be approved or not, based on the models.
McKinsey estimates that AI will deliver $13T in economic activity by 2030. So, when you look at what the market size of RPA is today — even though it’s growing significantly — we’re looking at a billion or two, and we’re merely scratching the surface. So, we have just gotten started on this journey in my experience. There are a tremendous number of efficiencies that will be gained by enterprises in the coming five to ten years as the AI technologies mature.
Let me put that in context. We have a customer — a large technology firm based here in Silicon Valley. They came to us to try and automate their order processing. They said they have tremendous radiance in demand, like many technology companies. More than 70 percent of their orders have come in the last few weeks. They have 50 full time employees processing orders. Even that number of employees was not sufficient to meet the year-end demand. They took our cognitive product — IQ Bot, to try and understand these orders, and extract information. Every vendor has a different format. So, it’s a very difficult task. In about six months, they have been live with us; they have automated, end-to-end, 40 percent of the order volume.
If you can think about it, if they have 50 full time employees — 20 FTEs that have been re-deployed to other work in just six months alone. In the first five weeks, they had attained ROI as they were able to automate close to 50 percent of the deal volume. And now they’ve gone to multiple times return on investment. So, hopefully, that sheds some light on how big the opportunity is, and how quick the ROI can be realized. That’s one enterprise example. In terms of realizing ROI, other firms might not be as quick if there are issues around change management within the company. It does depend on the organization; each one approaches it differently — but the opportunity is tremendous.
Executives might think that cognitive AI can do anything, and they think of highly complex processes to automate. That’s when we see the people get disappointed, and the projects fail, because you want to take baby steps in your cognitive journey. You’re doing RPA; this is the very next step. We recommend to people is pick high ROI processes - and invoices and POs are great way to start. You can explore Explanations of Benefits or Standard Settlement Instructions - the kind of documents we’re seeing that are really good places to start for people.
Then, grow from there and attack more and more. The key is to look at 80/20 rule - sometimes it’s 20 percent of the customers who generate 80 percent of the orders. Or it might be 20 percent of the vendors who’ll do 80 percent of the order volume. Go after the low hanging fruit and show high ROI quickly. Get by and then deploy it enterprise-wise.
If you’re further along on your cognitive technology journey — you’ll want to benchmark yourself against best-in-class companies. We have actually put together benchmarking tools that help enterprises benchmark themselves against how best in class enterprises are doing. We have strongly guided recommendations. If we’re truly to make this transformation successful, business and IT have to work hand-in-hand. IT needs to put the right level of controls, governance, and ensure the deployment is secure, and business needs to be empowered to build the bots on their own, to attain the velocity they need to drive the transformation.
We’ve seen iterative CoE’s work. First having a center of excellence, maybe for finance — then adding HR, then having a corporate-wide center of excellence that provides governance. Think of it as though enterprises need to be moving toward the app store model. The Google and Apple app stores are good models — where anyone can build an app or, in this case, it would be that anyone could build a bot.
But when your talent submits the bot — there is a process of governance they can look at, and say, “Hey, is the Bot secure? Does it meet our standards?” Then you have a quick turn-around of 24 hours to 48 hours to approve the bot and get it out into production. So, they have to move to that agile, app store-type model to get everyone to participate in the transformation. It’s about democratizing the digital transformation.
Walking through gateway of digital transformation
The state of the market is that some distinct patterns are emerging. The words Digital Transformation have been both extraordinarily ambiguous and remarkably ubiquitous in consultants speak. Now, they’re beginning to take some level of shape. What we’re really beginning to understand is that RPA - which is an incredibly useful harvester of tasks is an almost essential gateway to the more sophisticated digital transformation capabilities.
The state of the market is that there’s an emerging realization that most companies can’t “just go do” AI. Even to do IA, you probably don’t have what you need. People are becoming aware that they don’t have the right resources; they don’t have the right knowledge, experience, and infrastructure. This is a maturation point. For Intelligent Automation, we have crossed the Valley of Despair - as all new technologies eventually do - and we’re actually coming to the question now of “how do I scale this thing?” Increasingly, the conversations are moving to scale.
That means that for those that are awake and have a heartbeat, they are aware of this thing Called IA or Intelligent Automation (which includes RPA and RDA) - they have an aspiration to move to AI, and they’ve probably stalled somewhere. From a recent study of automation programs, we know about half of all the adventurers that set out, are stalled. They stall for a whole variety of reasons. The conversation is shifting now to ‘how do you scale? There are some very important changes that need to take place in how you’re running your program if you want to scale.
What we also know is that to enable intelligence in your automation program means you are needing to bootstrap a business-rules engine into your automation platform(s) and using that to drive some level of intelligence into the task orchestration. This is also a maturation point for the IA industry. As a set of products and competing manufacturers, they are all evolving. Customers too are evolving.
The early adopters have been doing this for three, four years, or more and are now practitioners. We have tinkerers — they’ve been doing it for a year or two, and they want to become practitioners. We have folks that are just at the pilot stage and finally those who just learned to spell RPA.
The answer to the question “what are you doing with your IA platform?” continues to be a crucial important question. What use cases are you solving? I firmly believe that intelligent automation platform software is literally a box of possibility. People look at me cross-eyed when I say that, “What does that mean?” Well, what it means is the R - in ROI - the return, is undefined. It can be zero or infinite. It totally depends on the business. Not IT, not anybody else, not your partner or consultant. It relies on how many use-cases the business has queued up to move into automation.
The costs of an IA program are largely people based, not the technology. Technology is by far the smaller cost when you begin to get into a scaled program. Large programs may have as many as 65 or 70 people doing discovery, configuration, and production management. Technology will be less than 10 percent of the total program cost. But another key cost is maintenance. It’s directly related to the issues around scaling. Robots don’t get sick, and they don’t go on vacation, but they do go on strike, and they don’t tell you why. They’re very sensitive to environmental change. Whether that’s process change or technology change, they’ve very sensitive to change. This is what I call a “Stall Point” – an inevitable challenge you will face when scaling. It is critically important you have a partner who can teach you how to detect and to manage environmental change at a level you’ve never managed before. Because once you’re far enough along, the sensitivity to environmental change begins to go up - I won’t say exponentially - but it’s not a linear curve either. The ability to learn how to detect that and manage it is, in a lot of cases, one of the primary Stall Points that programs will inevitably confront.
There are several Stall Points every program will face. Another is what I call TAPY or Total Automation Program Yield – that is, “all in”, what are we getting in return. Eventually the business will ask what the hard-dollar benefits are, so a well-managed program will always be concerned with some level of task harvesting for FTE savings and importantly measuring it with an approved methodology. This generates the core savings that pay for the parts of the program that are moving to strategic automation and eventually adding intelligence.
But once you move into IA - you’re now moving into a world in which you cannot proceed without IT at your side. BUT, with the right roles and expectations. You can do basic RPA with very limited support from IT. They need to provide you with infrastructure for the platform, security provisioning, and access management for the automation. The business can drive a very long way, with just that limited support. The conversations in the back-half of 2018 are shifting to, “Okay, so now I understand what it is, what it does, how it works, what is the right role of IT? What is the right role of audit?” I now understand these things. So, what does it take for my program to get over that exponential hill in front of me?
The role of IT very many changes, as you now want to bring pieces of real IT systems into your automation platform or program. Most enterprises begin the IA journey with a single platform - often an RPA platform. That is absolutely the right way to start. Harvest those tasks to pay for the more advanced stuff. Now you realize that you need to use advanced analytics to give your automation the smarts it needs to make a decision on whether or not to process a certain transaction, or fork left or fork right. Now you are asking to query live production systems for data to fuel intelligence and you will need IT with you for this.
Well, now you’re starting to change - you’re starting to move into this world of IA. It’s very rudimentary, but nonetheless, you’ve brought in some intelligence. It’s, “Hey automation, pick your head up, look. Is there a rock in your way? Or can you keep going straight? Basic automated decision making is the logical next step.
The manufacturers are starting to narrow the gaps in functionality. Some easing the connection to third party analytics and some offering “in-platform” machine learning capabilities. The market is realizing we need an easy start up, along with a pathway to advanced Intelligent Automation.
We’re also starting to see that the combination of multiple technologies is really where IA is achieving next-level success. There are some products out there that claim to deliver IA out of the box. I’m not aware of any production use cases I’ve seen that would convince me that that is a common occurrence. Not saying they’re not out there, just that I haven’t seen them first hand. There are advisors available that have truly been there and done that- and have five years more experience than you do - or six or seven years more experience than you do. I was recently talking to a very large services organization. They were proudly touting their top person as having two years’ experience. Trust me, they haven’t even gotten to the really hard Stall Points. We’re beginning to see some separation in terms of the kind of partners that you need to have to do this work successfully and well. You need somebody who’s actually run those traps before. Power point won’t do it.
I continue to believe and there is growing agreement, that RPA is the gateway to digital transformation. It really is. It is a way to get in, get started in a way that’s very consumable, low-threat, and low investment from a cost perspective. But it brings the organization to an important level of capability and awareness in a pretty short time - in as little as two years - to the point where they can now begin to invest in more sophisticated tooling, and then finally bringing intelligence into the automation platform. This is a non-trivial exercise. I can’t emphasize it enough. I’ve not seen anyone able to jump the basics. It’s like going to college when you didn’t go to highschool. VERY difficult to get a passing grade.
Particularly when you’re looking to disrupt work. It’s one thing to do a data science experiment, build a data model, get some predictive analytics, and then realize, “Well, that’s just a fascinating and cool intellectual exercise we have here.” You’re looking through a microscope and saying, “Hmm, that’s umm, really cool, but what do we DO with it?” As opposed to changing the way that 4,000 sales people work, or 4,000 wealth managers work, or 5,000 service delivery personnel work. Transforming work in a large enterprise is completely different than creating a machine learning model in a petri dish. For that, you will need the experience of a partner.
Applying RICE to budget planning
Thoughts on measuring IA affect on the enterprise
RICE is a prioritization framework shared by Sean McBride that works well in product management. As a product manager myself, I found that RICE can also help with prioritization of intelligent automation opportunities. During the intake process, the business provides information that estimates the Reach and Impact of the bot, such as time saved, cost avoided, or intangibles such as risk mitigation. The bot builder team defines the Confidence in delivering the bot on time as well as the Effort needed in terms of sprints. With this information, it becomes easier to compare automation initiatives across common metrics. So how do we apply this information once we have it?
Demonstrating value through past success builds trust and creates advocates that can help you in future engagements. RICE provides a mechanism to tie the results of your bot portfolio to your original business value forecasts to prove value and build trust as you go. The key is to provide value and build trust with the right people at the right time. Start by helping the finance and compliance teams to automate a few opportunities with high RICE scores. With this approach, you have proven value to influential teams that have a broad view of various processes within your organization, and the decision-making process used to fund upcoming initiatives. Leverage key wins with these teams to get a seat at the table for enterprise-wide budget conversations.
In the moment, order of magnitude estimations
Now, the calculus changes with regards to automation planning and budget. Since you have created automation advocates in the Finance and Compliance teams, you can leverage your relationship and their influence to get a seat at the table at those critical budget discussions. By utilizing a framework like RICE, you can apply the same justification for new budget requests and pick those opportunities where automation can add significant value. This enables you to provide an order-of-magnitude estimate in that moment and assess whether automation may be a better alternative to funding a new project. Since the value of automation has already been proven to Finance, they may be more open to suggesting a lower-cost bot as an alternative, freeing up capacity to fund a different project that may not have been previously approved for the budget cycle. Perhaps a regulatory agency is imposing new rules that require new funding to ensure compliance. Since the value of automation has been proven to Risk & Compliance already, they may be more open to funding a bot that can provide higher accuracy, can take on broader scope or can ensure compliance 24/7 instead of funding new resources.
With RICE, we have an ability to compare automation initiatives within a common framework, so we can prioritize automation needs accordingly. We also have a framework where we can compare automation alternatives to new budget requests and assess that in the moment of those critical budget discussions. By leveraging the RICE framework, we can provide exponential value to the enterprise by funding new automation where appropriate in the moment that matters most, budget season.
Building your team to tackle your unstructured data now
First, a few key principles to building your team to tackle your unstructured data:
• Realizing the actual dark/unstructured data itself
• Actually, finding AI talent (which is difficult)
• Showing the art of the possible or demonstrating value to the enterprise
• Proving an environment that is adaptable to change
Realizing the actual dark/unstructured data itself
Most companies are considering or have embarked on RPA, where best processes to robotize — with highest ROI are — high volume, standardized processes, reparative in nature and all have structured data. However, the majority of your data is unstructured. It’s that unstructured data which will lead to exponential growth.
Again - most companies are making business decisions based on structured data, which is a fraction of the total amount of available data. Your unstructured data refers to information that is not organized in a pre-defined manner.
The ability to access and make sense of your unstructured data will provide remarkably valuable information, which will propel the next level of automation. If companies and/ or teams can do this well, they will attain competitive advantage.
So, you have a data strategy?
It’s important to look at what these types of data essentially mean, where they come from, how they are used and, most of all, how they can generate value. Currently companies are mainly looking at structured internal data sources and have not started analyzing external data sources. External data sources are unstructured. Businesses need to know how to gather this information together and build from the knowledge contained within it. Even unstructured data has to be properly organized. There is a great deal of other important information hidden in unstructured data. This is where Artificial intelligence actually comes into play — as it can deal with all of this as unstructured data, which simply cannot be dealt with by a rule basis approach.
Actually, finding AI talent (which is difficult)
Organizations looking to attract AI talent are finding expertise scarce and costly. Most of the current talent in the workforce is at the big tech companies. The reality is that many companies — especially those outside tech industries — are still at the beginning of their journey.
And so, to simply remain ‘current’ we must be preparing for the future and determining our HR strategy if we want to build in-house or collaborate with external providers. In my company we value the AI intellectual capital but also want to understand the benefits. The big tech companies like Google, Amazon, and Microsoft have developed enterprise AI solutions — this makes the development of your own AI obsolete.
‘Big tech’ offers AI-as-a-Service such as MS Cognitive Services. If you accept their SaaS services, it may mean you do not require a heavy AI developer but rather a quality IT person/ developer that can apply these solutions. But even these people are in short supply.
We have educated our HR department and business partners to define future talent profiles. Together with HR we have created a network of recruitment agencies and educational institutions. We have created reverse mentoring to ensure we find internal bottom-up talent.
How familiar is the organization and how ready is the staff for this AI technology? We all require change management capabilities throughout the enterprise. Each organization must have someone who evaluates the organization’s AI maturity while simultaneously understanding the change culture. We need talent who can explain — in simple terms — what AI can do, and not do- and what that means for the current staff. This talent needs vision, network, communications skills, bridge tech and business while retaining a competitive overview.
Showing the art of the possible or demonstrating value to the enterprise:
There are still very few practical AI examples. Those out there come at a cost, which makes your ROI still quite difficult in a small-scale project. Similar to RPA the use of pilot programs is recommended. The same principles of RPA apply to AI - go simple, fast, low cost and with a narrow scope. Be able to show the art of the possible via a demo. Using existing AI software can be effective, as it helps your enterprise build skills and learn as it goes. The AI services of Google, Amazon and Microsoft enable small-time developers in AI, without the upfront costs or hassle-which make them a safe bet. Another way to go about this is to ask yourself, ‘can you collaborate with business development, R&D, etc. departments?’ They traditionally will focus more on customer facing parts of the business and have more advanced solutions than backend parts of the business. And if you can’t collaborate, steal with pride.
While AI is your toolkit for unstructured data, AI is defined broadly and can be deployed in many different ways. Before you do anything in this area ensure the following:
• Clearly defining how AI can solve both immediate and future problems is an imperative.
• Having a clear value proposition/business case is vital to any data-driven initiative, especially one involving the collection and management of unstructured data sets
• Marketing, customer satisfaction, healthcare and supply chain all apply
• For finance there are many opportunities: â—‹ Advanced analytics to use unstructured data will provide more attributes to enhance knowledge-based processes and sense making: credit decisions, risk analysis, budget process, better forecasting and assist with judgment calls. â—‹ There is a real opportunity to become more forward looking- in conjunction with RPA it will mean next level of automation.
• Eventually finance will also use Conversational Interfaces, Microsoft Cognitive Services provides Language Understanding (LUIS) there could be a PoC there.
• Last but not least, try to use as much AI assistants AI in your own devices (e.g. Siri, Alexa) to trigger ideas of the art of the possible…
• You might find that AI can be used to enlighten customers and business leaders
Proving an environment that is adaptable to change:
A digital strategy is often characterized by the application of new technologies to existing business activity and/or a focus on the enablement of new digital capabilities to their business… (source Wikipedia).
The same is applicable to AI and similar to RPA. Larger corporations of course are having the discussion of whether it should develop from IT or from business function, where it directly supports the processes. A CoE (IT or Business) is a good place to start because often they have an agile, nimble development framework and experience with PoC’s.
Take the incremental approach with AI as it will be a safe environment to experiment. The expectation is also that RPA vendors will broaden their scope to AI so this will help an easier transition. This will lead to more rapid development and higher AI tech adoption. CoE’s are a good place for ideation. Again, the right place to start is not in technology, but in the business cases that you want to test.
A three-part interview on the nature of artificial intelligence
Rajeshwari Ganesan is the Associate Vice President and Head of Engineering of Nia Knowledge – Infosys Nia, Infosys’ AI platform. She shares the advancements in the area of machine learning with language processing, vision-based computing, and bio-inspired architecture with compensatory networks, parallel neural pathways, which mimic the human brain and mind.
It’s Raji’s history with technology, which has enhanced her ability to take us all to an AI informed future. She says, “I’ve seen technology evolve from mainframes to client-server systems prior to 1999. Post that it was web-based systems - then it became distributed computing and now we have AI and machine learning, both of which are dominating every aspect of computing.”
Each of these step-change technologies in her past have essentially educated her about the vastness of capabilities and possibilities. She adds, “AI and ML are going to be ubiquitous. With machine learning, computers can program themselves instead of having to be programmed by us. ML methods look at the data we generate, they figure out what we do to set their goals”. Therefore, setting purposeful goals is the key.
Computing in the mainstream has been leveraged primarily for analytical and logic-based operations
Raji, as she is fondly called, explains how AI actually works. “Today, if you look at computing systems, they are extremely good in doing logical operations, repetitive tasks at high speed, which works well and augments what humans aren’t naturally good at. But imagine a scenario where machine intelligence could mimic biological human intelligence. It could be language learning, perception or process of learning, or even the fuzziness we demonstrate, which makes us survive and evolve in adverse and new environments. Even a simple objective of making machines understand the context and semantics of natural language or its generation is a non-trivial task – machines’ intelligence can still not fathom it. So instead of being disappointed, how can we still leverage machine learning as it continues to evolve is the key question.
Every word we speak becomes a multi-dimensional vector
How do machines understand the language? Diving back into how it works, Raji explains, “the way human brain processes, our neocortex understands the context and semantic language is entirely different from the way machines understand the language. So, under the bonnet of the machine, what we see is a lot of numbers for each word. A famous scientist once said, a word is worth a thousand vectors for the machines. Every word we speak becomes a number and multidimensional vector – a word embedding. This form of representation of language makes language computable. We can use this representation to find similarity between words or understand the context. This technology is applied to contracts analysis system, wherein it enables machines to understand contentious clauses in legal documents, redline or highlight them. This way machine aids humans to ensure contracts are done quickly.
The human mind has compensatory networks
Saving time, cutting cost, improving efficiency are some of them. With these abilities, AI can take the leap. “For instance, assume a person with visual disabilities. As the human mind has compensatory cognitive abilities, this person’s hearing becomes very developed, which compensates for the vision he or she doesn’t have. This ability to have compensatory perception is the way to counter the deficiency”. Today, if we really look at the language processing of machine learning, it’s not where it exactly ought to be. There are deficiencies, but the question is whether these deficiencies could be offset? Raji and her team are taking inspiration from the human brain to create their architecture. She says, “Today when we read a document, our vision precedes our understanding of the text. So, we see it first then read and comprehend. In case of a machine too, by adding the visual perception prior to language can help it better understand the context of what is being read. With time, there would be more and more networks, which would provide parallel neural pathways of different abilities, which humans are currently doing- the sum total of which can achieve a certain objective.” Functioning of the brain is so complex and not very well understood by the most talented neuroscientists. Raji explains, “I’ll give you another example, which I encountered a few days back. I had a conversation with my daughter, and I just blew it up. I stepped back and said to myself, ‘Can I explain myself as to why I responded in a certain way?’ Simultaneously, I was trying to make my machine learning models explain themselves. Sometimes, when they make a certain prediction, it cannot be a black box. The machines need to explain themselves and I was trying to put it through as architecture. And I realized, it’s a lot easier for me to write those algorithms in machines to explain themselves rather than trying to reason why I was behaving in a certain way.”
The circular ecologies and the age of technology ignorance and hypocrisy
“The future belongs to those who believe in the beauty of their dreams.”
~ Eleanor Roosevelt
I was always driven by my dreams. Be that the case, when I sat to write this piece, I realized that for the past few years it wasn’t dreams that shaped my perceptions but frustrations. We tend to think about frustrations in a negative context, yet I try to let it define the outcome of my everyday activates after all frustrations are born out of bad experiences and frictions, and if I am aware of that I can do something about it.
When talking to people, I tend to quote the New York Times from 1920: “A rocket will never be able to leave the earth’s atmosphere.” Just 25 years later that feat was accomplished. The ensuing 50 years brought incremental achievements. It wasn’t that we didn’t succeed in soundly shuttering that prediction but did need to wait for Elon to say “screw it” to understand that leaving the earth’s atmosphere is but a small piece in the puzzle of building a sustainable civilization.
Listening to Carl Sagan talking about the Voyager, I can’t but think why, whenever we refer to NASA, the first relation is to the moon landing. I do not, even for a second, underestimate the magnitude of the impact of Neil Armstrong’s “That’s one small step for a man, one giant leap for mankind” statement had on humanity. Yet I do feel we capitalized on that as a one-off PR stunt, and from a technology point of view, the moon landing was nothing, but incremental modifications NASA made to rockets so they can land a man on the moon.
The Voyager, on the other hand, also sent a man-made object to space, yet the objective was not technological by nature but philosophical – what are we going to do about aliens once we encounter them? How do we push forward to explore the universe while — without refueling — maintaining and still capturing data from an object that continuously increases its distance from earth?
Only when we confront the unknown will we excel beyond imagination
The amount of collaboration needed to pull off the Voyager was unprecedented at that time- and I will argue that even today, that collaboration is unique. The results are amazing. The Voyager left our solar system and continues to explore space. And while my $1K smartphone loses its reception every time I go into a basement, the Voyager is still sending images back to earth.
The Voyager project was never about technology, it was about exploring the unknown, and I believe that only when we confront the unknown will we excel beyond imagination.
What are the fundamental questions we need to ask ourselves when facing yet another unknown? Not in space, but the change technology is going to enforce on us?
The hidden change
Humanity is facing an unprecedented change in its perception of reality and in the narratives that defined its existence. Much as past extinctions that pushed the reset button for the entire planet — thus enabling rejuvenation and new creation — humanity is but a touch away from such a reset.
Since the invention of the axe, technology was always the force that paved the way for better and smarter social constructs. From Watt’s steam engine in 1775, sewage systems, elevators, and shipping containers — to screens, smartphones, processing power, and machine learning — it is technology which helps us to push the barriers and build our world. It’s the one function that never stops, it continually changes itself, reinvents and defines new horizons — most, unfortunately, are now beyond our ability to understand.
The industrial revolution wasn’t just a buzzword, it was the compounding accumulative impact of humanity’s maturity and acceptance that met with technology on the same field of understanding and mutual benefits — values. Yes, it bolstered urbanization, innovation, and creativity for many years, yet it is fast approaching a critical peak and is about to leave us facing the unknown, naked from knowledge.
The ideas of our future are now anchored in code
For the past few years, a new breed of technologies is stepping into our arena. Artificial Intelligence, robotics, genomics, biotechnology, nanotechnology, terotechnology — adjustable reality and the codification of value interactions enable us to reexamine and repurpose every aspect of our existence. From our digital-selves to the cities we live in — from mobility, energy, and communication to new financial models — the ideas of our future are now anchored in code.
The future always required strategic, patient thinking and to be honest, before the 50s, these were qualities we possessed. Most books, art or movies written and produced had a holistic view of things. They describe a point in time where the world was dominated and powered by technological gadgets, body enhancements, artificial intelligence, autonomous cars, flying cars, cities in the clouds or underwater but most important they investigated the impact of these ideas of society.
We outsourced our potential innovation and creativity and started to build technology for the sake of technology
Sometime around 1983 - 1984 computation power shifted humanity’s focus from imagining to engineering. Suddenly we had machines that could do the things we always did but better and faster and we became addicted to them. We enslaved our minds. We outsourced our potential innovation and creativity and started to build technology for the sake of technology.
We replaced our strategic vision with a tactical search for answers, we have surrounded ourselves with buzzwords like “the 4th industrial revolution,” “IoT,” “smart cities” and more — all while forgetting that technology was meant to be nothing but a means to an end.
Focusing on the means, we are now left behind and unable to see the end. It’s time to rethink life by imagining the potentials and the desired impacts not only from a technological point of view but also incorporating ethics, morality, trust, and education into this equation.
The stupid intelligence
Without a point of reference that is anchored in past experiences, it’s hard to tell the future.
Hawking dismissed the ideas that the existence of the universe can be attributed to a single point in which everything that happened before is meaningless. Yet I cannot ignore the fact that we did evolve from that point of the big bang and we do not carry the heritage of things that existed before that peak.
Throughout the years we have struggled to understand intelligence. Although organic and inorganic matters are both made from the same building blocks we call atoms — we still cannot breathe life into silicon. Maybe, intelligence is the narrative that manifests itself via the bonds and reciprocal relationships in the creation of a self-contained universe. Perhaps it is not a stand-alone “brain” but the fact that that brain is derived from the complexity and context that pushed that brain into existence. We have created beautiful technologies but as we cannot break our “anchored in historical chains” perspectives while searching for life on other planets, we cannot “build” intelligence by merely copying existence into a code, so we are left with machines that operate in the realm of life yet are not alive.
It was not long ago that innovation boomed from the ideas of individualism, self-interest, logic, reputation, and honesty. And by all accounts, life was good. It seemed that we have found the formula for economic growth. But a strange thing happened. Alan Greenspan, in the aftermath of the collapse, said: “I made a mistake in presuming that the self-interest of organizations, specifically banks and others, was such that they were best capable of protecting their own shareholders.”
The bigger mistake was that — even so — all data showed almost no growth in productivity, the government sided with the 0s and 1s that continued to push numbers to new, unimaginable heights. We assumed that the “room can speak Chinese,” and we were dazzled by the output. We had a perfect “distance economy,” and we missed the fact that John Searle’s “Chinese room” experiment (this is the moment where you open a browser and Google John Searle’s “Chinese room”) showcased the simulation of intelligence rather than possession of intelligence.
Let us examine this for a moment. Throughout history, the success of intelligence systems depended on the idea that situations should be driven by their potentials and values are determined via the beneficial reciprocal relationships between the actors that make the system. In Searle’s demonstration — the wished outcome — the connections between the actors, was mechanical and without any reciprocal value.
In the model of the economy, we took this yet one step further- we placed a singular actor to define the inputs, outputs and the value creation mechanisms. At each point of action there was a different view on the model.
It was not the model itself that failed, it was the way we implemented it.
Awakening - The divided world
The most significant risk we have as a society is not from the unknown, but for the known to fall apart.
For the first time in history (at least the one we can trace back to the big bang) natural evolution had peaked it’s potential - there is no place to go from a biology point of view. Yes, we might be a bit faster and jump a bit higher, but we have reached a point where our organic structure just cannot evolve anymore. Even with genetic modifications — sooner or later — we will hit a limit. It is not only our organic structure that is facing evolutionary ends; our mental abilities are also severely limited. This is also translated from the narratives in which we created the reality around us — from geopolitics and global C-Suites — we managed the world with a limited understanding of the challenges ahead and thereby under-utilize our evolutionary potential in almost every area.
The fundamental building blocks of society can be folded into three domains, mobility, energy and communication. Traditionally, they always operated with degrees of freedom. Yet, they never applied that to the reciprocal relationships between each other. Today, and because of technological development, these connections can manifest themselves via the codification of the logistic systems that carry the societal interaction models — yet instead of letting this natural process evolve itself — we are confining it to the limited information input/output model of yesteryear’s society and are therefore leaving ourselves with output patterns from our halcyon days.
When the sovereign know less about the needs of a free market than the players that must compete in that market, the value creation is twisted by bias. The role of the sovereign is to create a set of societal APIs that will define its own operating boundaries and at the same time will be open, so the market can repurpose itself to its own benefits.
Time to change
It is hard to write about changes without talking about politics and leadership. It is said that if you do not accept change yourself, you will be rendered irrelevant and eventually perish.
Change is one of the most laborious processes to swallow- as individuals and moreover as a society that flourishes on the idea that what exists is right and shouldn’t be changed. Thought fixation defines the reciprocal relationships between the various examples that we use to measure success — but they also make us forget that by doing so, we build a wall around our creativity and innovation.
Some may disagree, but facts point to the notion that for the past ten years the global economy as a whole and Silicon Valley, in particular, presented a slow yet constant decreased ability to innovate. Most companies (excluding some well-documented examples) have reached their “golden age,” or in other words, they got old, less observant, full of bloated ego but more importantly — they became fragile. They are stuck in the loop of thought fixation and to ease the pain, they have surrounded themselves with rings and rings of regulations that are anchored in paper-based politics.
The ideas that our knowledge and values are inductive yet must be shaped by the deductive nature of reality — so they can deliver value — is a great way to look at businesses, society and politics operating together. It is not about what was, nor about what will be, but what is.
“Looking into the future, you cannot predict which groups will survive, it’s well known that many advanced groups eventually fade away.”
~ Charles Darwin, Origin of Species, 1859
We live in an unfortunate reality where many leaders have dismissed their responsibility for the future and lost their appetite to think big. These heroes without vision are busy analyzing and planning, while others are building for immortality. Powered by siloed vision — they are fragmenting our reality and creating a new circle of ideological wars.
In the current claimant of blind leadership, we have to distance ourselves from the contradictions that the political situation is placing in front of us and look at change as an opportunity to use our collective mind.
Not to continue on the same path of “things we know that we know” but disconnect from the laziness and fear and build an infrastructure for new business models, new definitions of innovation and most importantly create a mindset, that will encourage the next change.
We need to believe that the best solutions to a problem are not hitting it with everything we have, but by viewing it from all possible angles. If something does not work for its intended purpose, it might work for something completely different — that is the meaning of change.
Also read: AI & Bots in banking and finance indutries