How can your enterprise benefit from machine learning right now?
A proof of concept case study
Add bookmark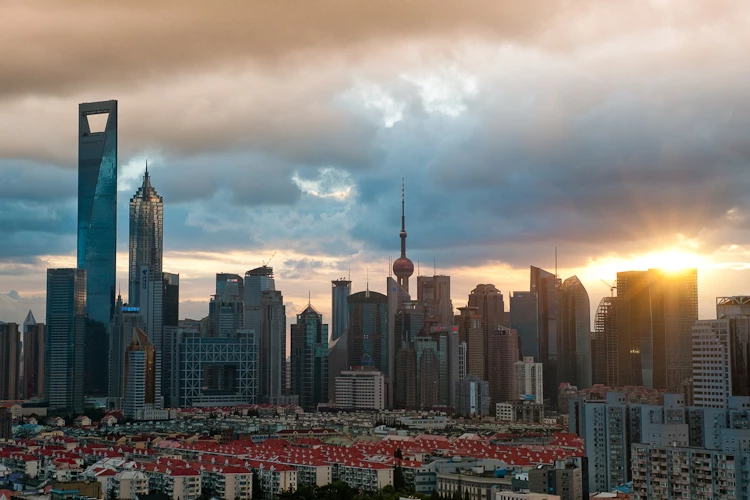
Discover why a leading bank deployed machine learning in trade finance to address rising operation costs and growing regulations and compliance.
Trade Finance is one of the most overwhelmingly complex functions comprising several stakeholders from seller to buyer, insurance parties, seller’s and buyer’s bank, logistic partners and regulatory authorities which warrants for compliance at each step. Each trade transaction processes close to 12 to 13 documents, 100 pages with more then 5000+ data interactions.
It’s predominantly paper-intensive and prone to error due to structured and unstructured data obtained from multiple internal and external sources. The manual nature of this function for gathering, matching and auditing data from each of the parties makes the process even more time-consuming.
The bank was looking for a scalable and agile trade finance operations model however the disparate and highly manual systems were acting as blockades to operational efficiency and also exposing the bank to regulatory risk.
The challenges :
The bank highlighted the following challenges:
- Eliminate manual maker roles in data entry of export documents
- Reduce rework in export document collection and discrepancy management process
- Control the rising operations cost
- Automate OFAC compliance checks
- Improve customer experience through faster processing of trade documents
The solution :
Download this proof of concept case study to discover how Hexaware helped the bank overcome these challenges, enable digital innovation in Trade Finance and positively impacted the customer experience by helping to create relationships with greater value for both parties.